The Chinchilla compute optimal point for an 8B (8 billion parameter) model would be train it for ~200B (billion) tokens. (if you were only interested to get the most “bang-for-the-buck” w.r.t. model performance at that size). So this is training ~75X beyond that point, which is unusual but personally, [Karpathy] thinks this is extremely welcome. Because we all get a very capable model that is very small, easy to work with and inference. Meta mentions that even at this point, the model doesn’t seem to be “converging” in a standard sense. In other words, the LLMs we work with all the time are significantly undertrained by a factor of maybe 100-1000X or more, nowhere near their point of convergence. Actually, [Karpathy] really hope people carry forward the trend and start training and releasing even more long-trained, even smaller models.
Karpathy seems to be saying that if we have better compute, we can train up models to a more ideal level for better AI and AI performance.
Congrats to @AIatMeta on Llama 3 release!! 🎉https://t.co/fSw615zE8S
Notes:Releasing 8B and 70B (both base and finetuned) models, strong-performing in their model class (but we'll see when the rankings come in @ @lmsysorg :))
400B is still training, but already encroaching…— Andrej Karpathy (@karpathy) April 18, 2024
If a large language model is undertrained by 1000 times, it means that the model has not been trained on a sufficient amount of data or for a sufficient number of iterations to reach its full potential. In other words, the model has not learned enough from the data to perform well on the tasks it was designed for.
To illustrate this, let’s use an analogy. Imagine you’re trying to learn a new language. If you only study for 10 minutes a day, it will take you much longer to become fluent than if you studied for 10 hours a day. Similarly, if a large language model is trained on a small dataset or for a short period of time, it will not be able to learn as much as it could if it were trained on a larger dataset or for a longer period of time.
The performance of a large language model is often measured in terms of its perplexity, which is a measure of how well the model predicts the next word in a sequence. A lower perplexity score indicates better performance. If a model is undertrained, its perplexity score will be higher than it could be if it were trained properly.
The amount of improvement that can be achieved by training a model properly depends on a variety of factors, including the size of the model, the quality of the data, and the specific task the model is being trained for. However, in general, it is possible for a model to achieve a significant improvement in performance if it is trained properly.
For example, a recent study found that increasing the size of a large language model from 1.5 billion parameters to 175 billion parameters can lead to a 10-fold improvement in performance on some tasks. This suggests that larger models can be more powerful than smaller ones, but only if they are trained properly.
In summary, if a large language model is undertrained by 1000 times, it means that the model has not been trained on a sufficient amount of data or for a sufficient number of iterations to reach its full potential. If the model were trained properly, it could potentially achieve a significant improvement in performance.
AI’s Red Pajama dataset from Oct/2023 continues to hold the crown with 30 trillion tokens in 125 terabytes. Notably, all major AI labs have now expanded beyond text into multimodal datasets—especially audio and video—for training frontier multimodal models like Gemini, Claude 3 Opus, GPT-4o, and beyond.
What is in one of the major 5 trillion token (20-30 Terabyte) text AI training datasets?
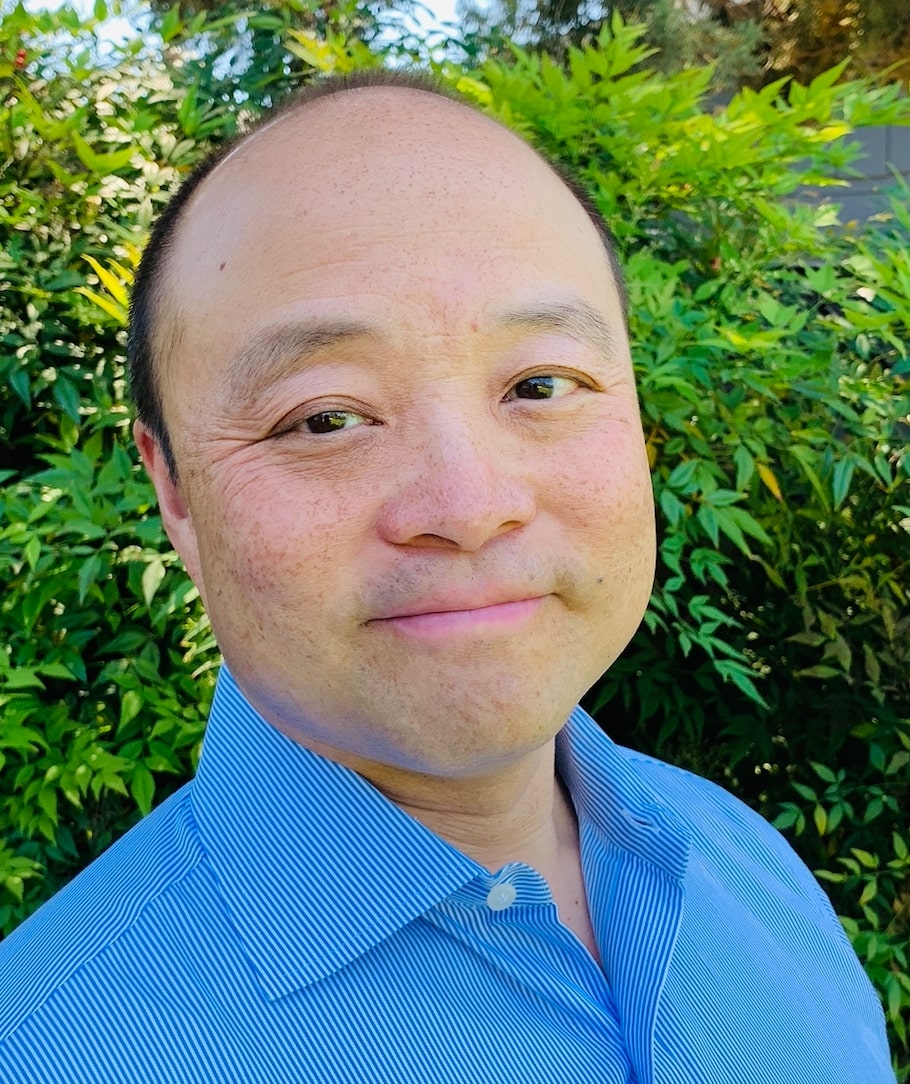
Brian Wang is a Futurist Thought Leader and a popular Science blogger with 1 million readers per month. His blog Nextbigfuture.com is ranked #1 Science News Blog. It covers many disruptive technology and trends including Space, Robotics, Artificial Intelligence, Medicine, Anti-aging Biotechnology, and Nanotechnology.
Known for identifying cutting edge technologies, he is currently a Co-Founder of a startup and fundraiser for high potential early-stage companies. He is the Head of Research for Allocations for deep technology investments and an Angel Investor at Space Angels.
A frequent speaker at corporations, he has been a TEDx speaker, a Singularity University speaker and guest at numerous interviews for radio and podcasts. He is open to public speaking and advising engagements.
Great insight Brett. I’ve heard talk about the use of Synthetic Data to train models at an accelerated rate. But reality is messy and chaotic. But actual biologically evolved intelligence is able to operate well in the messy real world. To me it seems that some ineffable quality that resides in the realm of tacit knowledge and whatever lies beyond it, may be missing in the models. Especially in something that is the brainchild of so many ( I suspect) highly functioning autistic tech bros, wo are neuro-divergent in a way that does not necessarily embody the full richness of human wisdom, which transcends mere intelligence. Just my 2 cents worth.
I’m ‘neuro-divergent’ myself, Asperger’s, and I didn’t need a billion man-years of training to exhibit intelligence. Generally speaking, ‘neuro-divergences’ that don’t result in being institutionalized aren’t missing much.
I think the issue is that the LLM’s really are just highly evolved predictive models, like a very fancy auto-complete, they don’t yet embody concepts. Real intelligence needs the ability to form concepts.
We’ll know that they’ve actually cracked AI when the AI training data is comparable in volume to the sensory input of 10-20 years of human experience. Not that you wouldn’t still want more than that, (No reason your AI shouldn’t be equipped with a lot larger knowledge base than a human can accumulate.) but it should be enough to emulate human level intelligence.
In fact, I’d say the more intelligent an AI, the LESS training data it should require, because a key element of intelligence is the ability to efficiently learn. And current ‘AI’s’ are horribly inefficient at learning.
“… I didn’t need a billion man-years of training to exhibit intelligence…
We’ll know that they’ve actually cracked AI when the AI training data is comparable in volume to the sensory input of 10-20 years of human experience…”
I’m not sure why people do not realize that humans and other animals have the benefits of millions and millions of years of training built in from birth. It’s called survival. Think of a baby giraffe. It walks in minutes. It doesn’t really learn this. It’s wired in. It’s only calibrated, The circuit is already there, and so it is with humans. The “key”, it’s hardwired and we are only calibrating it.
“…Molecular evidence suggests that the ability to generate electric signals first appeared in evolution some 700 to 800 million years ago.” Wikipedia
Language appears to have a framework built in, it must be trained, but the framework is already there. All sorts of image processing, already there. Walking, balance, framework is there already. I suggest there is millions of years of pre-wired training. I don’t think AI’s have the same number of neurons as a brain. They can go faster but look at the number of values a neuron can take. It’s got to be way higher.
“…The human brain has some 8.6 x 10^10 (eighty six billion) neurons. Each neuron has on average 7,000 synaptic connections to other neurons. It has been estimated that the brain of a three-year-old child has about 10^15 synapses (1 quadrillion)…” Wikipedia
Most AI’s today have a few months of training. It’s nothing.
Combine that with specialized neural network hardware, evolved over hundreds of millions of years. Our puny silicon processors are woefully underpowered and under trained.
This seems obvious to me. I wonder why people much smarter do not seem to see this? Maybe I suffer from a huge Dunning-Kruger effect, but the facts seem fairly obvious, and the numbers are…what they are.
I think a great deal of intelligence is wired in, and we only go about calibrating it. The brain, I believe, is just a huge batch of hardwired neural tricks layered on top of each other. I’m not saying there’s no learning, only that the unlaying hardware has already been set up with lots of efficiencies when we start.
I’m not sure that I’m actually going to disagree with you, as such. I’m just going to say that, if you have to replicate our entire evolutionary history to emulate intelligence, then you haven’t invented artificial “intelligence”, you’ve invented artificial “biology”.
Artificial “intelligence” would start out at about newborn baby level before the training. Not flatworm level.
This really illustrates that LLM ‘ai’ isn’t really intelligent, but instead is just a glorified exercise in hyperdimensional curve fitting. If you need a billion times the data that a human takes in during their entire lifetime to properly emulate human intelligence, what you’ve got is clearly NOT actual intelligence. It’s hardly more than a really big, compressed look up table at that point.
Is there even enough data out there to do a thousand times the training? With the data already being contaminated by the output of “AI’s” pretending to be humans?
I’m not saying LLM’s don’t have utility. Rather, they’re demonstrating how much utility you can get without actually achieving intelligence.