NVIDIA DGX-2 is a server rack with 16 Volta GPUs and Dual Xeon Platinums for $399,000. It packs a total of 81,920 CUDA Cores with 512 GB HBM2 memory and a 14.4 TB/s aggregate bandwidth and 300 GB/s GPU to GPU. The total power consumption of the rack is 10,000 watts and weighs 350 pounds.
DGX-2 provides 10X the processing power of DGX-1 of six months ago, unveiled in September 2017.
It’s $399K for the world’s most powerful computer. This replaces $3M of 300 dual-CPU servers consuming 180 kilowatts. This is 1/8th the cost, 1/60th of the space, 18th the power.
AlexNet, a pioneering network that won the ImageNet competition five years, has spawned thousands of AI networks. What started out with eight layers with millions of parameters, is now hundreds of layers with billions of parameters. The growth is 500x in five years. Moore’s law would only have suggested 10X.
The fastest supercomputer in world is 125 petaflops, fastest in U.S. is 100 petaflops. And this is 2 petaflops.
Nvidia has launched the new Quadro GV 100. It is based on the advanced Volta GPU architecture. Quadro GV100 packs 7.4 TFLOPS double-precision, 14.8 TFLOPS single-precision and 118.5 TFLOPS deep learning performance, and is equipped with 32GB of high-bandwidth memory capacity.
GV100 sports a new interconnect called NVLink 2 that extends the programming and memory model out of our GPU to a second one. They essentially function as one GPU. These two combined have 10,000 CUDA cores, 236 teraflops of Tensor Cores, all used to revolutionize modern computer graphics, with 64GB of memory.
In less than a decade, the computing power of GPUs has grown 20x — representing growth of 1.7x per year, far outstripping Moore’s law.
In just five years the number of GPU developers has risen 10x to 820,000. Downloads of CUDA, our parallel computing platform, have risen 5x to 8 million.
Nvidia announced a new version of the TensorRT inference software, TensorRT 4. Used to deploy trained neural networks in hyperscale datacenters, TensorRT 4 offers INT8 and FP16 network execution, cutting datacenter costs up to 70 percent, Huang said.
The software delivers up to 190x faster deep learning inference than CPUs for common applications such as computer vision, neural machine translation, automatic speech recognition, speech synthesis and recommendation systems.
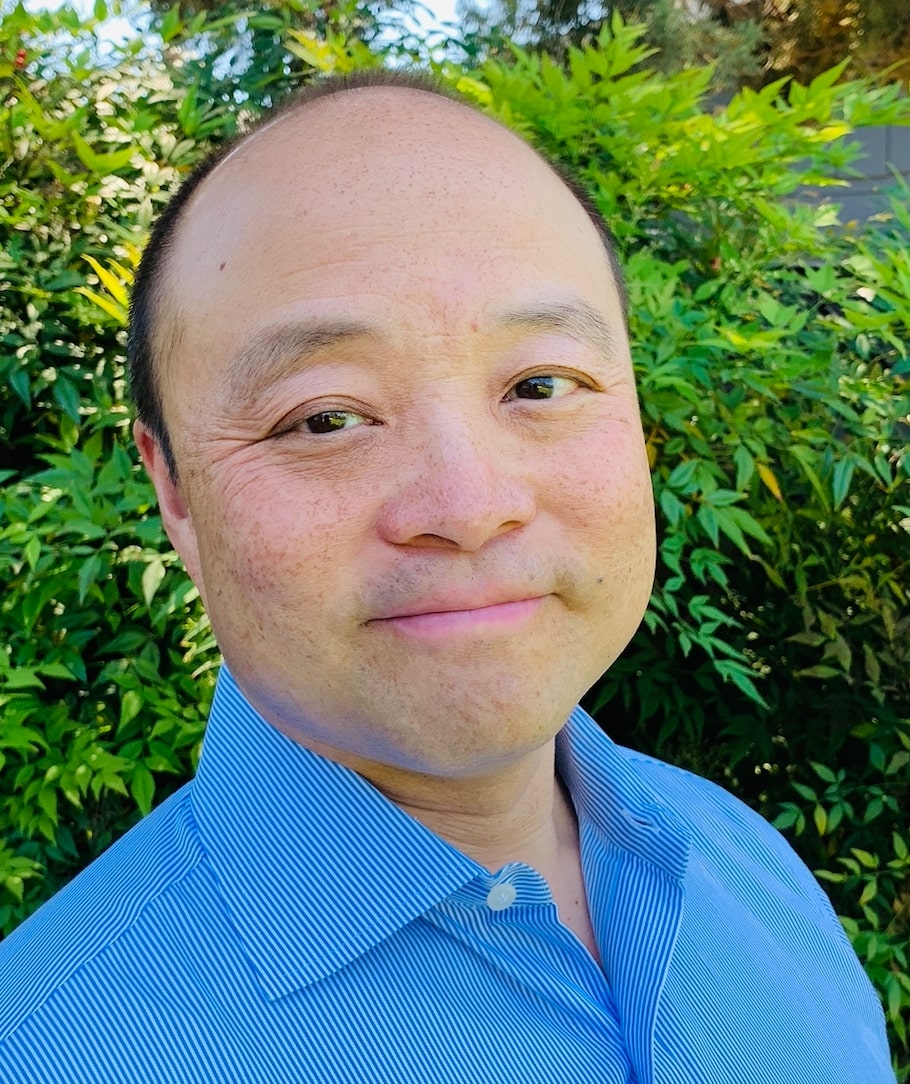
Brian Wang is a Futurist Thought Leader and a popular Science blogger with 1 million readers per month. His blog Nextbigfuture.com is ranked #1 Science News Blog. It covers many disruptive technology and trends including Space, Robotics, Artificial Intelligence, Medicine, Anti-aging Biotechnology, and Nanotechnology.
Known for identifying cutting edge technologies, he is currently a Co-Founder of a startup and fundraiser for high potential early-stage companies. He is the Head of Research for Allocations for deep technology investments and an Angel Investor at Space Angels.
A frequent speaker at corporations, he has been a TEDx speaker, a Singularity University speaker and guest at numerous interviews for radio and podcasts. He is open to public speaking and advising engagements.