Nvidia had $6.12 EPS and revenue of $26 billion which beat ($5.59 estimate on EPS) on EPS and revenue. Revenue estimates were $24 billion.
NVIDIA (NASDAQ: NVDA) today reported revenue for the first quarter ended April 30, 2023, of $7.19 billion, down 13% from a year ago and up 19% from the previous quarter.
GAAP earnings per diluted share for the quarter were $0.82, up 28% from a year ago and up 44% from the previous quarter. Non-GAAP earnings per diluted share were $1.09, down 20% from a year ago and up 24% from the previous quarter.
“The computer industry is going through two simultaneous transitions — accelerated computing and generative AI,” said Jensen Huang, founder and CEO of NVIDIA.
“A trillion dollars of installed global data center infrastructure will transition from general purpose to accelerated computing as companies race to apply generative AI into every product, service and business process.
“Our entire data center family of products — H100, Grace CPU, Grace Hopper Superchip, NVLink, Quantum 400 InfiniBand and BlueField-3 DPU — is in production. We are significantly increasing our supply to meet surging demand for them,” he said.
During the first quarter of fiscal 2024, NVIDIA returned to shareholders $99 million in cash dividends.
NVIDIA will pay its next quarterly cash dividend of $0.04 per share on June 30, 2023, to all shareholders of record on June 8, 2023.
Outlook
NVIDIA’s outlook for the second quarter of fiscal 2024 is as follows:
Revenue is expected to be $11.00 billion, plus or minus 2%.
GAAP and non-GAAP gross margins are expected to be 68.6% and 70.0%, respectively, plus or minus 50 basis points.
GAAP and non-GAAP operating expenses are expected to be approximately $2.71 billion and $1.90 billion, respectively.
GAAP and non-GAAP other income and expense are expected to be an income of approximately $90 million, excluding gains and losses from non-affiliated investments.
GAAP and non-GAAP tax rates are expected to be 14.0%, plus or minus 1%, excluding any discrete items.
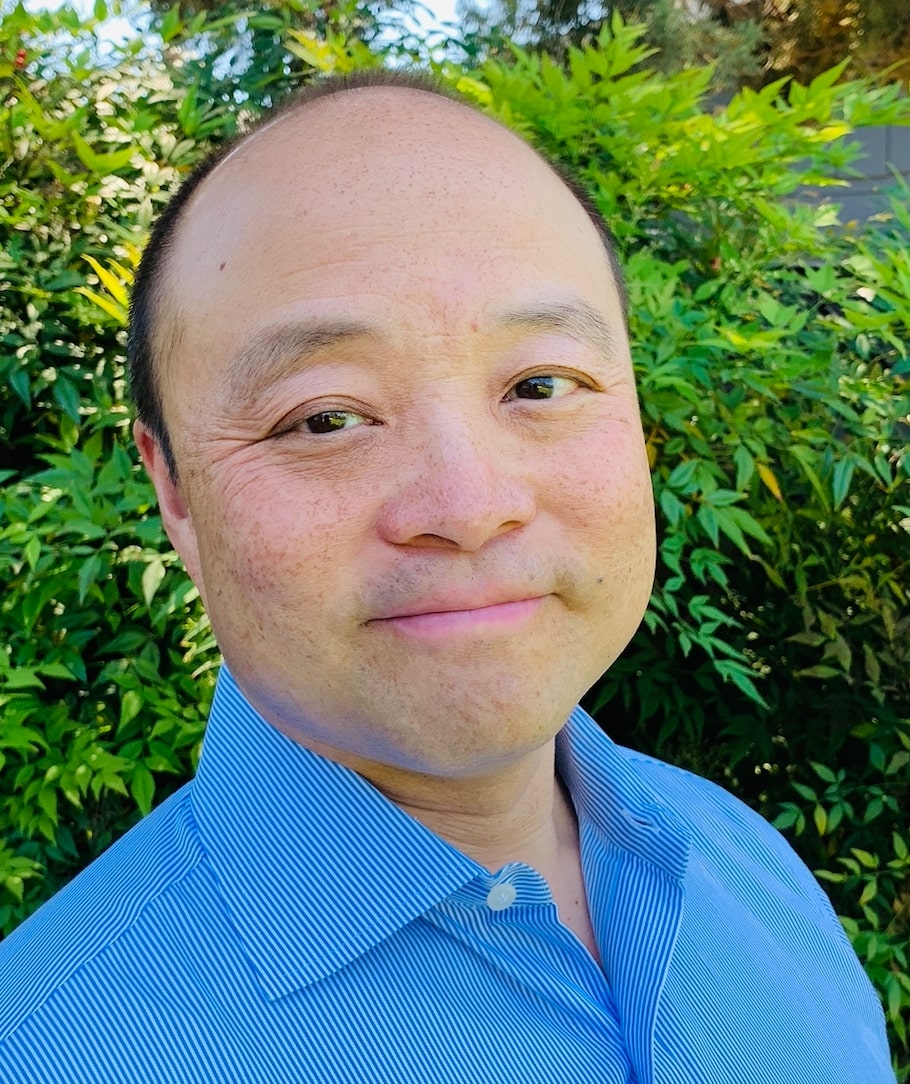
Brian Wang is a Futurist Thought Leader and a popular Science blogger with 1 million readers per month. His blog Nextbigfuture.com is ranked #1 Science News Blog. It covers many disruptive technology and trends including Space, Robotics, Artificial Intelligence, Medicine, Anti-aging Biotechnology, and Nanotechnology.
Known for identifying cutting edge technologies, he is currently a Co-Founder of a startup and fundraiser for high potential early-stage companies. He is the Head of Research for Allocations for deep technology investments and an Angel Investor at Space Angels.
A frequent speaker at corporations, he has been a TEDx speaker, a Singularity University speaker and guest at numerous interviews for radio and podcasts. He is open to public speaking and advising engagements.
It’s possible the Nvidea competitor is already here.
Here are 5 competitors to Nvidea: https://www.nanalyze.com/2020/11/ai-chip-startups-nvidia/
This 3+ year old article refers to Groq (not Grok) which now claims its Language PRocessing Units (LPUs) are cheaper and 10X faster than any other AI chip, including Nvidea’s, due to latency issues. Says Groq itself: https://groq.com/ –
“Why should I care about fast inference?
Fast inference is crucial in many applications, and here are some reasons why you should care:
Improved User Experience: Fast inference enables faster response times, which can significantly improve the user experience in applications like:
Chatbots and virtual assistants: Faster response times lead to a more seamless and engaging experience.
Recommendation systems: Quicker inference times enable more personalized recommendations, improving user satisfaction.
Search engines: Faster inference allows for more accurate and relevant search results, enhancing the overall search experience.
Increased Scalability: Fast inference enables you to handle a larger volume of requests, making it ideal for:
High-traffic websites and applications: Faster inference allows you to handle more concurrent requests, reducing latency and improving overall performance.
Real-time analytics: Faster inference enables real-time analysis and insights, making it suitable for applications that require rapid data processing.
Cost Savings: By reducing the time it takes to perform inference, you can:
Reduce the number of servers needed: Fewer servers are required to handle the same workload, resulting in cost savings.
Optimize infrastructure: Faster inference enables you to optimize your infrastructure, reducing the need for expensive hardware upgrades.
Competitive Advantage: Fast inference can be a key differentiator in competitive markets, allowing you to:
Outperform competitors: Faster inference times can give you a competitive edge, enabling you to respond faster to user requests and provide a better user experience.
Attract and retain users: A faster and more responsive application can attract and retain users, leading to increased customer loyalty and retention.
Improved Model Performance: Fast inference can also lead to:
Better model performance: Faster inference enables you to train and deploy more complex models, which can lead to improved accuracy and better decision-making.
In summary, fast inference is essential for improving user experience, increasing scalability, reducing costs, gaining a competitive advantage, and improving model performance. By prioritizing fast inference, you can create a more responsive, efficient, and effective application that sets you apart from the competition.”
This 3yo article refers to $300m raised by ex-Google employees for Groq: https://www.forbes.com/sites/amyfeldman/2021/04/14/ai-chip-startup-groq-founded-by-ex-googlers-raises-300-million-to-power-autonomous-vehicles-and-data-centers/
Groq claims its chips can process 1 quadrillion operations/second. This is way beyond human comprehension, but the possibility of the company’s success isn’t, as this much more recent article will attest to: https://venturebeat.com/ai/ai-chip-race-groq-ceo-takes-on-nvidia-claims-most-startups-will-use-speedy-lpus-by-end-of-2024/
Local TV said that Nvidia spend 20% to 30% of their outgoings each year on R&D. Compare that with companies run by MBAs just looking for a quick profit.
Can’t believe I actually went kinda heavy on this before it blossomed. Wish I’d gone heavier but, then again, wish I’d had money to invest when I tripped across Amazon in 1998 and thought: “I should really get some of this even if they aren’t making money.” Alas, some sort of natural law at work here.
Diamond mine for Nvidia. Tech companies buying Ai optimized gpu’s on massive scale. Nvidia smart enough to spend some on research and dev so others can’t catch up and they keep huge margins.