If Large Language Models debate their answers they can reach better answers. A complementary approach to improve language responses where multiple language model instances propose and debate their individual responses and reasoning processes over multiple rounds to arrive at a common final answer. The findings indicate that this approach significantly enhances mathematical and strategic reasoning across a number of tasks. They demonstrate that the approach improves the factual validity of generated content,reducing fallacious answers and hallucinations that contemporary models are prone to. The approach may be directly applied to existing black-box models and uses identical procedure and prompts for all tasks they investigate. The findings suggest that such society of minds approach has the potential to significantly advance the capabilities of LLMs and pave the way for further breakthroughs in language generation and understanding.
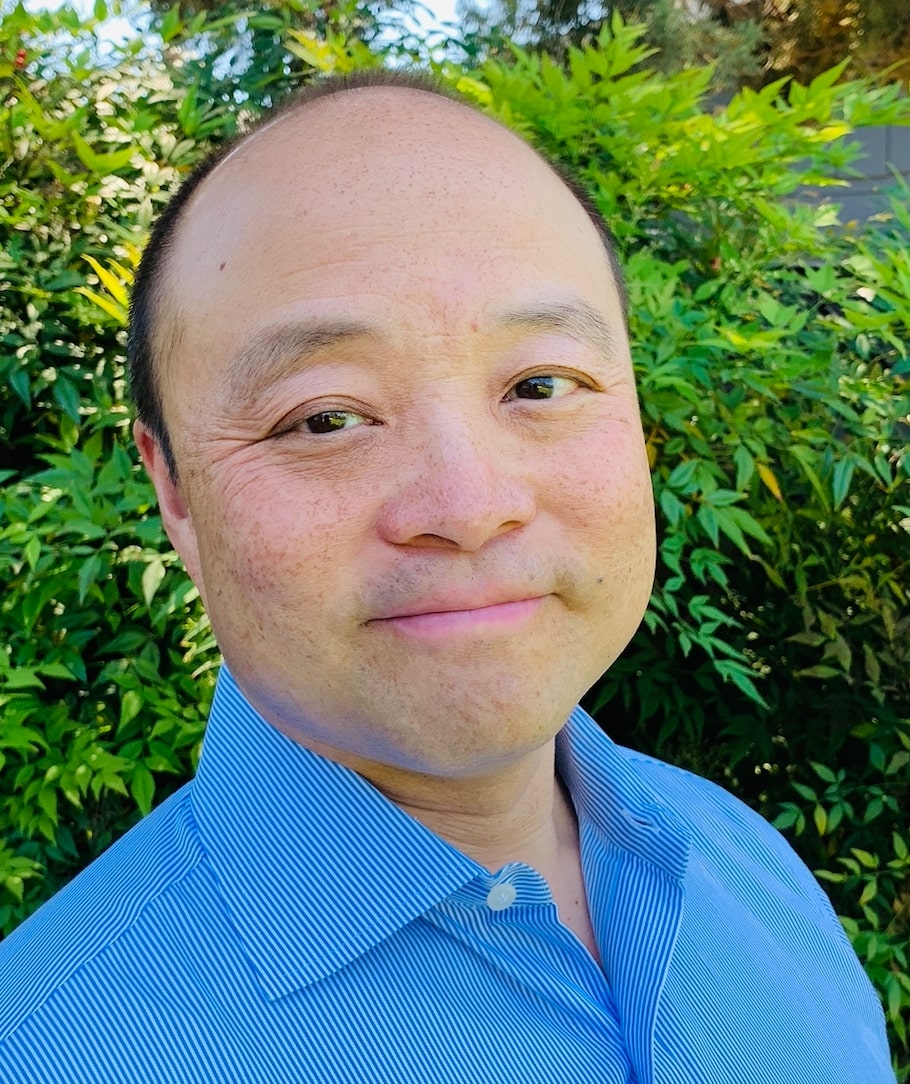
Brian Wang is a Futurist Thought Leader and a popular Science blogger with 1 million readers per month. His blog Nextbigfuture.com is ranked #1 Science News Blog. It covers many disruptive technology and trends including Space, Robotics, Artificial Intelligence, Medicine, Anti-aging Biotechnology, and Nanotechnology.
Known for identifying cutting edge technologies, he is currently a Co-Founder of a startup and fundraiser for high potential early-stage companies. He is the Head of Research for Allocations for deep technology investments and an Angel Investor at Space Angels.
A frequent speaker at corporations, he has been a TEDx speaker, a Singularity University speaker and guest at numerous interviews for radio and podcasts. He is open to public speaking and advising engagements.
Math, as a way of expression, has an absolute conclusion as to what it try’s to “say”. Language is much more nuanced. The same sentence, in the same language, said with different inflexion on certain words, can convey different “intent” from the person saying it. Math has an “absolute conformity”, that is universal. That’s why we call it math. Language? Much more fluid, adaptable, shall I say; “squishy”? I say: “Oh, please don’t do that, please” as opposed to saying the same words but in the way: “OH, PLEASE DON’T DO THAT, PLEASE!!!” Same words, but convey different meaning, that the consequences of what I say may be one thing, or something else. Just a thought.
I’ve been saying this for a while. A single output from an LLM is a single thought in a human – but we don’t do this. We rarely publicly submit the first thing that pops into our minds, we have an internal process of filtering and reprocessing.
To reach a high quality of output, LLM’s have to be so structured as well. It’s really obvious when you consider how we think ourselves.
That said, LLM’s are seriously limited in that they don’t learn in inference mode (which for a user of an LLM is all the time). So until LLM training mode can be made cheap enough to run, they will always be limited to what they already know and they’ll remain incapable of learning from their own processing.
This papers shows why chatgpt and bart are not worth the usertime, mostly they are wrong. Other ai do much better i asked them the paper questions, stock vales are overpriced
I’ve never known a committee decision better than talent, education, and experience of a single man.
Yes, but these aren’t humans. They have no ego nor second agendas. They just see at the chain of thought and come with some additional feedback and conclusions the first pass didn’t made.
The closest equivalent would be brainstorming with passably talented and cooperative people.
It strikes me that math is about the last domain, short of straight symbolic logic, where debate should be required. Intuition might might be helpful in achieving a quick result, but verifying the result should be objective, not subjective.
Do AI’s not “check their work”?
And this is “grade school math”, not anything advanced. It’s about the most mechanical level of math around.
This really underscores that the AIs are not actually engaged in reasoning, don’t actually comprehend the subject. Because you’d expect any human who actually had internalized the rules of math to routinely get 100% accuracy at grade school math.
LLMs do mistakes and hallucinate, having a second or third opinion is more likely to form a consensus that is closer to truth.
They’re actually hallucinating 100% of the time, essentially, and the hallucinations are carefully crafted to statistically correlate with the input data well enough that most of the time they happen to agree with it.
But if they actually understood the math they were doing, they could check their results to see if they made sense, the way grade school students are expected to, and correct it if the result was a mistake.
Without understanding, all they can really do is try to improve the fidelity of the hallucinations, in order to drive that correlation up a bit, and do multiple runs to vote on which hallucination was most frequent.
When they actually get understanding, you’ll see that error rate take a sudden step to zero without requiring exponentially increased resources being involved.